Artificial Intelligence (AI) has permeated every aspect of business and our personal lives. A report from McKinsey estimates that around $13 trillion of global economic activities will be driven by AI in 2030. Given today’s unprecedented openness between research institutions and industries, it is exciting to see what AI-related discoveries will be brought to life by this collaboration.
At the Image Information Systems Laboratory (headed by Prof. Masahiro Iwahashi) at Nagaoka University of Technology (NUT), the team of Prof. Ryosuke Harakawa works on various AI applications that uniquely address real-world social challenges.
Prof. Ryosuke Harakawa of Nagaoka University of Technology. Photo credit: Nagaoka University of Technology
To date, they have come up with AI for polishing cutlery, monitoring flood control, and providing road maintenance support.
“When you try to solve problems that are social and environmental in nature, and to some extent global in scale, there is a great need to bring individuals with highly specialized skills, knowledge, technical knowhow, and resources to the table,” he begins.
“The AI applications that we have come up so far wouldn’t be made possible without the collaboration that we had with different departments at the university, government agencies, partner organizations, and other experts,” he continues.
The Impact of AI: By the Numbers
13
70
30
Notes from the AI frontier: Modeling the impact of AI on the world economy (McKinsey Global Institute, September 2018). Read the report here.
The laboratory and their research projects
At the laboratory, Prof. Harakawa works in a team of 18 individuals, comprised of Ph.D. candidates, master and undergraduate students. The team specializes on the use of image recognition, social media / data mining, deep learning, and big data analysis to develop AI and IoT technologies.
While AI has been applied to bring business intelligence, automate business process, and deliver a novel approach to customer interaction, the lab focuses on multimedia signal processing for AI-related applications that bring value to society.
01. AI for Polishing Cutlery:
A Collaboration with Hardware Technology
Tsubame-sanjo in Niigata, Japan has been producing cutlery for more than one hundred years and is known internationally. The said industry dates back to the Edo period but due to the aging and falling population, necessary manpower to support the process of polishing cutlery has seen a sharp decline.
Some cutlery made from Tsubamesanjo and was once used at a Nobel Prize Award banquet that follows the ceremony. Photo credit: Gideon Franklin.
“To help sustain the production of these regional products, our team worked with NUT’s Department of Electrical, Electronic and Information Engineering, Prof. Ohishi (an expert on robotic engineering), the regional company of mechanics, and the Ministry of Economy, Trade, and Industry (METI), to develop an AI technology to support the craftsmen in polishing cutlery.”
“To make this happen, we developed a new method to determine the shape of the cutlery, and adopted a hue-based coding method to ensure a more accurate polished product.”
“We used cameras and projector to obtain a 3D surface measurement of the material, and robots to polish the spoon and fork based on a hue-based coding method.”
Y. Takimoto, R. Harakawa, and M. Iwahashi, “Hue-based gray coding method for three-dimensional surface measurement of cutlery with specular reflection,” IEEE Trans. Circuits and Systems for Video Technology (TCSVT), pp. 1-10, 2020. Photo credit: Iwahashi Laboratory, NUT.
The existing method of determining the shape of the cutlery is based on luminance captured by the camera.
“Specular reflection, which is the mirror-like effect seen from the surface of cutlery, can serve as cue for the shine, glossiness, or finish of a cutlery. But the specular reflection causes the performance degradation in the existing method for a 3D surface measurement based on luminance.”
“With a hue-based coding method, we can accurately measure the 3D surface for enabling us to judge the finish of the cutlery,” Prof. Harakawa explains.
02. AI for Flood Control and Road Maintenance:
A Collaboration with Civil Engineering and Disaster Prevention Science
Nagaoka and Niigata are home to the longest and widest river in Japan, the Shinano River. At 367km in length, the river surrounds many communities and has been extensively used for electricity generation and irrigation.
Despite the benefits, seasonal high rainfall can result to flooding which can claim lives, damage properties, and destroy crops such as rice, which is the principal product produced in the region.
“Using data mining (from weather news, Twitter, and social media), image processing obtained from river surveillance cameras, and deep learning, we analyze river images to accurately detect flood risks. This collaboration project with Prof. Matsuda at the Department of Civil and Environmental Engineering and the company related to the disaster prevention system can contribute to ensure the safety of the residents of Nagaoka and Niigata.”
・T. Oga, R. Harakawa, Y. Matsuda, and M. Iwahashi, “Correlation analysis of cyber-physical data for flood disaster prevention: Case of typhoon Hagibis,” in Proc. IEEE Global Conf. Life Sciences and Technologies (LifeTech), 2020, pp. 109-110.
・K. Kasuga, R. Harakawa, and M. Iwahashi, “Classification of in-vehicle camera images for guardrail inspection support,” in Proc. IEEE Global Conf. Life Sciences and Technologies (LifeTech), 2020, pp. 113-114.
・T. Oga, Y. Umeki, M. Iwahashi, Y. Matsuda, “River water quality estimation based on convolutional neural network,” in Proc. APSIPA Annual Summit and Conference, 2018, pp. 1305-1308. Photo credit: Iwahashi Laboratory, NUT.
“Our deep learning technology is also used to enable road maintenance support for human labor. In-vehicle cameras allow us to capture the condition of roadside guardrails and highway fences. And through deep learning, we can classify the degradation level and make a recommendation if we need to replace or repaint.”
03. AI for Plasma Simulation:
A Collaboration with Applied Physics
In the realm of applied physics, Prof. Harakawa’s team has developed an image processing technology that is capable of observing ultrafast phenomena such as the molecular processes in plasmas.
“Plasmas are hard to observe because, like gases, they do not have fixed shape or volume, and have less density than solids or liquids. Also, because they are ultrafast phenomena, they cannot be observed in existing image capturing devices.”
M. Tsumori, S. Nagai, R. Harakawa, T. Sasaki, and M. Iwahashi, “Contour-adaptive image restoration based on compressed sensing for ultrafast phenomena,” Applied Physics Letters, vol. 117, no. 4, pp. 044102-1–044102-6, 2020. Photo credit: Iwahashi Laboratory, NUT.
“Our image processing technology can combine overlapped images, and restore ‘real images’ at each time. This collaborative research with Prof. Sasaki at the Department of Electrical, Electronics and Information Engineering can accelerate the plasma simulation or any phenomenon that exceeds the frame rate.”
”Most of what we have come up so far are based on interdisciplinary researches and on a close collaboration between software and hardware infrastructure. Collaboration is very important to make these projects successful.
Prof. Ryosuke HarakawaAsst. Professor, Nagaoka University of Technology
The team’s research on image processing technology for plasma simulation and hue-based coding method for polishing cutlery were both accepted for publication in the top journal in applied physics and electrical and electronic engineering, respectively.
Prof. Harakawa’s initial foray into AI
Prof. Harakawa was originally interested in multimedia information retrieval and recommendation. When he first started the research, social media such as YouTube and Twitter were popular and widely used.
“During that time, terms such as “big data” and “unstructured data” (untagged data such as image, video, and music) attracted wide attention especially in the research community. User-generated media came from different sources. And the space where these data exist was very heterogeneous and unstructured.”
“The problem that time was, if you are going to input queries that are not suited based on the design of the algorithm, then, you are not going to see relevant results.”
To acquire the desired contents based on the query, Prof. Harakawa’s team developed a model that uses consensus clustering of data based on semantic and sentiment similarity estimation.1
EFFECT #1:
With unstructured data, it is difficult to understand important information because various topics are scattered. Through AI, we can classify keywords via semantic understanding of big data.
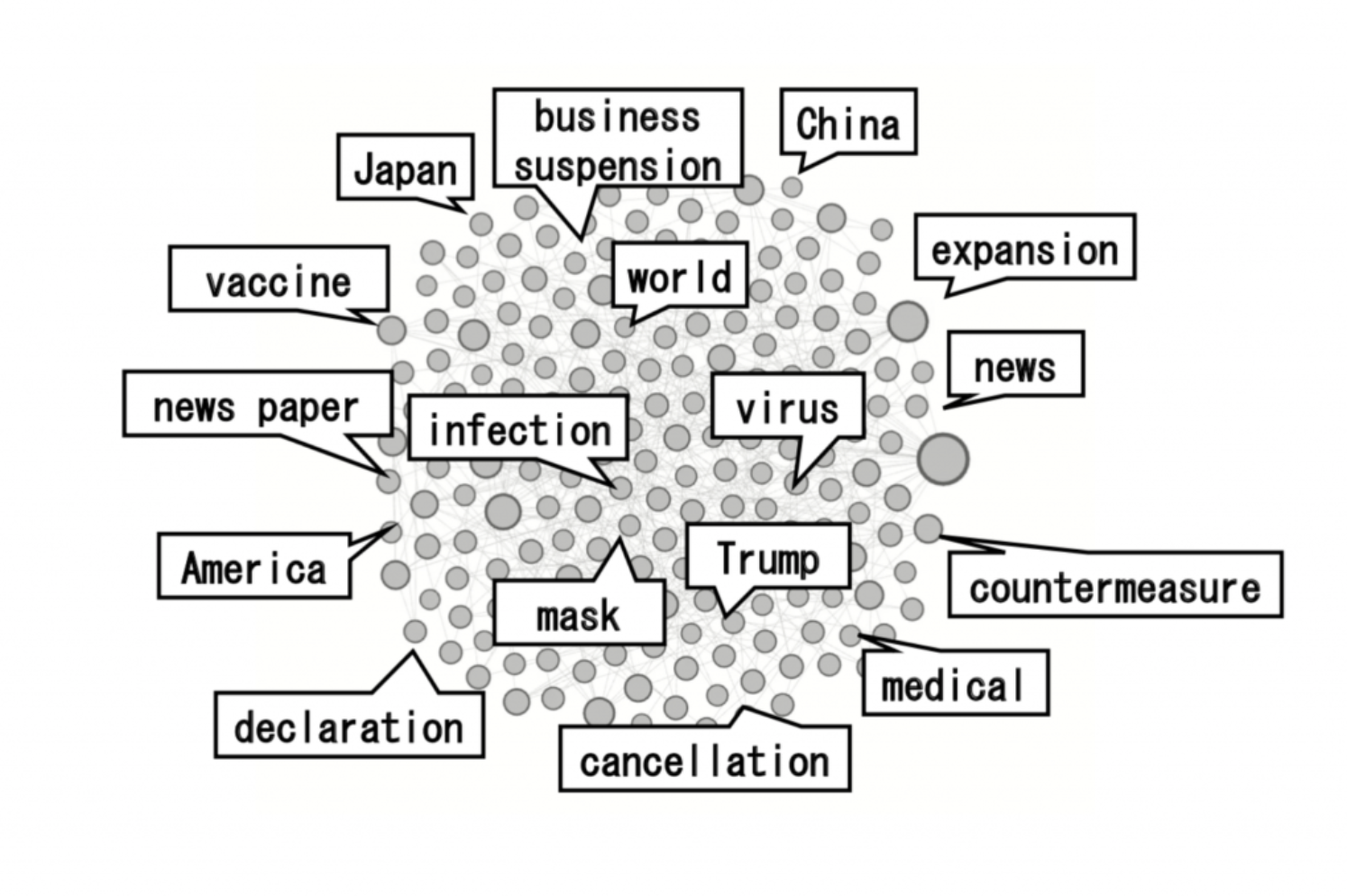
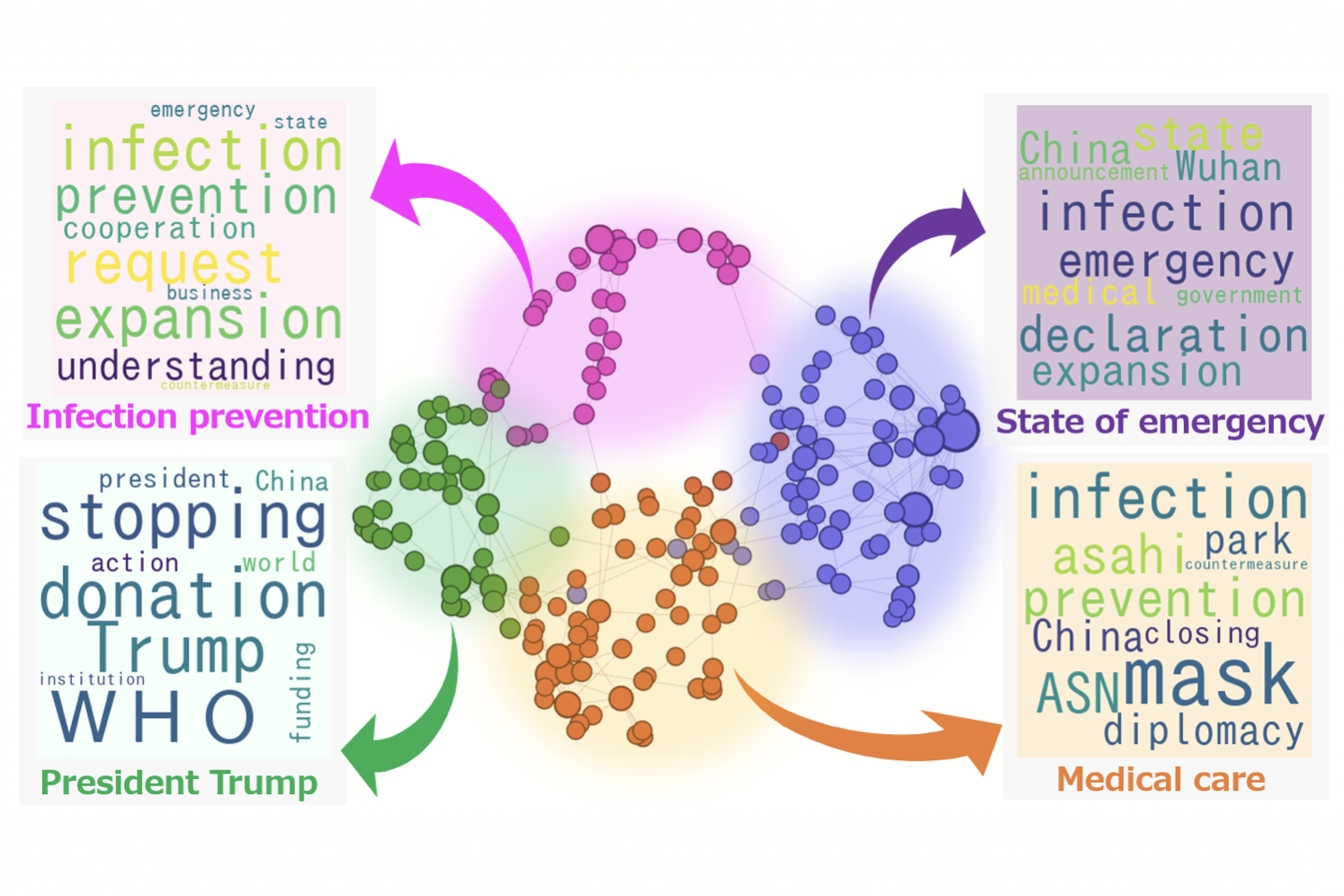
原川 良介, 岩橋 政宏, “ツイートコミュニティの中心性算出 —新型コロナウイルス感染症に関わる重要語抽出への応用—,” 第23回 画像の認識・理解シンポジウム (MIRU), vol. IS3-1-3, pp. 1-4, 2020. This is a result of extracting typical topics on Twitter from April 1 to April 15, and does not support any particular theory or opinion. Photo credit: Iwahashi Laboratory, NUT.
“Our model efficiently analyzes these unstructured data in order to come up with the hierarchical structure of similar content groups. This helps us extract useful information for the user from a sea of ambiguous data,” he explains.
“In sentiment estimation, we look at the emotions or attitudes expressed in a text. I think the easiest example would be classifying a text as something that is ‘positive’ or ‘negative.’ The word love for example can be viewed with a positive sentiment, while hate is viewed, otherwise. Now, when you look at other words that show the same sentiment, that’s when you have sentiment similarity.”
How Prof. Harakawa sees the future of AI
From Prof. Harakawa’s field of expertise and area of research at the laboratory, he considers that AI can become the controller of ‘information flood.’
“Information flood happens when a user of the Internet gets a sea of information, which causes the user to become unable to process all the data that is presented to a user.”
Using COVID-19 as an example, he further explains:
“In unprecedented incidents such as COVID-19, we’ve seen how a lot of information and misinformation (ex. 5G network being the cause of COVID-19) were spread in the cyberspace. As a result, users cannot acquire the necessary knowledge from the Internet, and some people acted inappropriately in the physical space.”
“This is because we can only remember knowledge that we originally knew, and cannot discover new knowledge in the unprecedented scene by the current technology. We developed the AI to overcome this limitation.”
In Prof. Harakawa’s research, the team looked at Twitter and identified keywords that are highly correlated to the number of infections of COVID-19 as the knowledge.
”In the COVID-19 era, AI can become really impactful for controlling information flood and giving insights to human actions in the cyber-physical spaces.
Prof. Ryosuke HarakawaAsst. Professor, Nagaoka University of Technology
“By automatically understanding the semantics of many tweets and extracting similar tweet groups, people can discover new knowledge efficiently. And at the same time, we can visualize the minority opinion hidden in the majority. In a way, we can see the value of AI when it comes to avoiding human bias.”
EFFECT #2:
We can further classify keywords in detail and can discover hidden information.
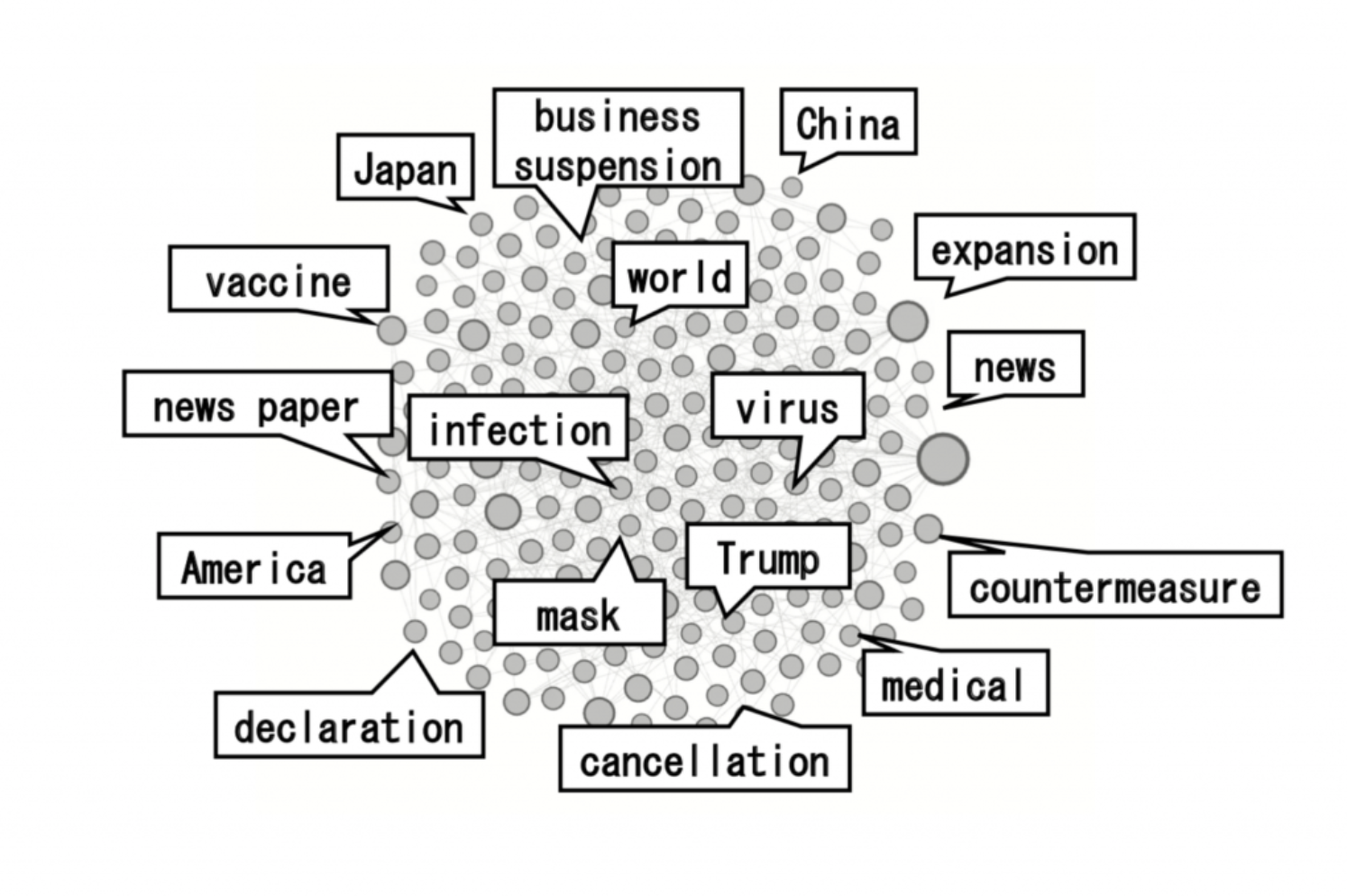
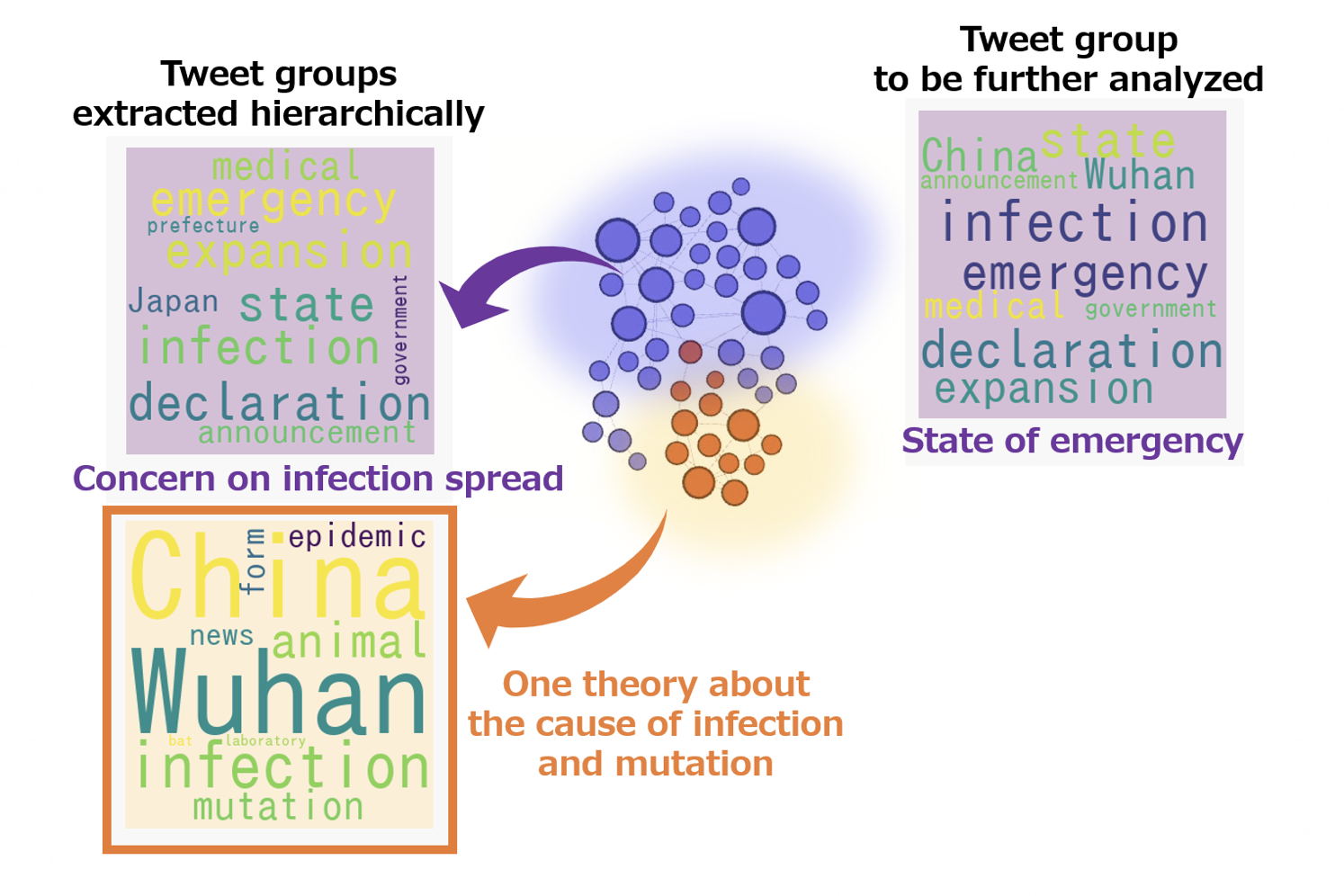
原川 良介, 岩橋 政宏, “ツイートコミュニティの中心性算出 —新型コロナウイルス感染症に関わる重要語抽出への応用—,” 第23回 画像の認識・理解シンポジウム (MIRU), vol. IS3-1-3, pp. 1-4, 2020. This is a result of extracting typical topics on Twitter from April 1 to April 15, and does not support any particular theory or opinion. Photo credit: Iwahashi Laboratory, NUT.
Message to incoming students of AI
“Current students at NUT have a lot of potential, and I have seen many students who are good at quickly implementing software and hardware programming. However, many of them do not know their own potential and do not have the confidence.”
“In the future, I would like to meet students who are patient and have the willingness to challenge known and unknown experiences. Through the fundamental researches and collaboration with other fields and partners such as industries, companies, and/or government, I would like to make them aware of their own potential.”
“And eventually, through their own research, I look forward to seeing them obtain significant research achievements be it in world-class top journals or research that has practical, real-world applications,” he concludes.
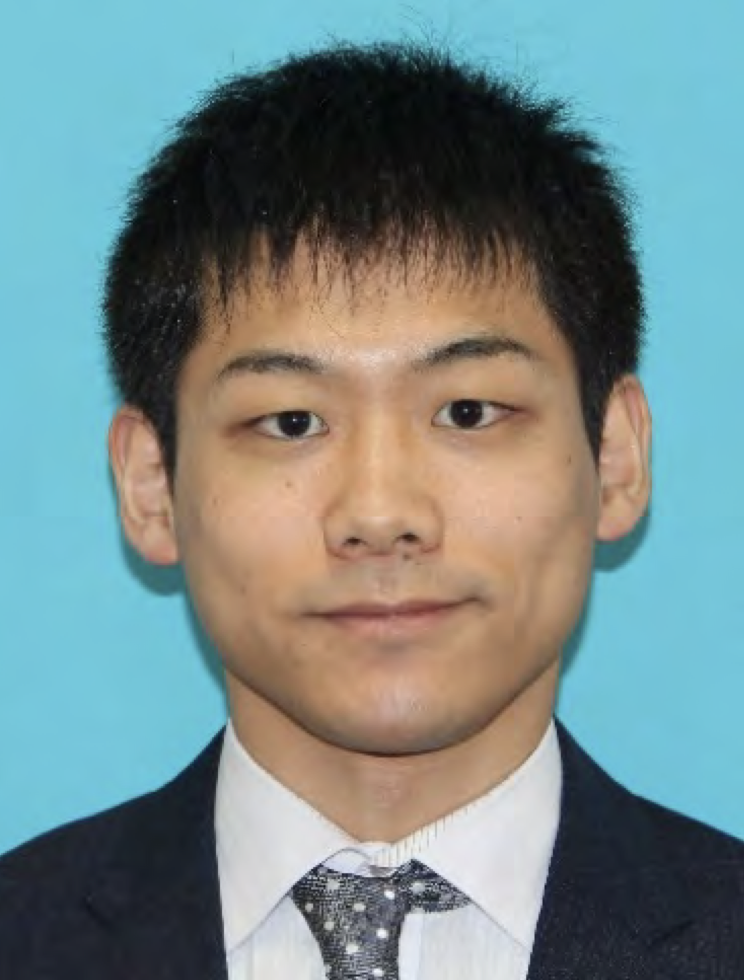
Brief Bio
Prof. Ryosuke Harakawa
Prof. Harakawa is an assistant professor at the Image Information System Laboratory of Nagaoka University of Technology. He holds a Ph.D. from Hokkaido University and specializes in the fields of multimodal semantic understanding, complex network, Web and SNS data mining, and information retrieval / recommendation, and visualization.
Stay connected
1 R. Harakawa, S. Takimura, T. Ogawa, M. Haseyama, and M. Iwahashi, “Consensus clustering of tweet networks via semantic and sentiment similarity estimation,” IEEE Access, vol. 7, pp. 116207-116217, 2019.
Main image by Nagaoka University of Technology